Authored by Zeksarias Bassa
Abstract
Adoption of improved food crop technologies is known to be the prerequisite for productivity improvement. However, due to different socioeconomic, demographic and institutional factors the level of food crop technology adoption and utilization is not optimal. A meta-analysis is performed to review empirical estimates of Adoption factors of improved food crop technologies in Ethiopia. The result indicated the adoption of the technology defined by sample size, technology type, study period. The analysis result also confirmed that the mean size effect of food crop technology adoption estimate is function of training, irrigation, extension service and credit access, oxen holding, cooperative membership, TLU, labor force and income. This implied that through awareness creation, improving farmer to farmer and farmer to extension and research linkage, improving credit access, infrastructural development, livestock ownership and income earning opportunity, it is possible to accelerate the speed of food crop technologies. The study results also justified that food crop technologies only focused on the specific technology type and quantity, not on how the technology implemented by farmers, these assumed to be probable reason for low adoption of improved practices that resulted in low agricultural production and productivity the sector.Keywords:Adoption; Food crop; Means size effect; Meta-analysis; Ordered Logit; Technology
Introduction
Modern agricultural technology defined and summarized in different major categories such as application of optimum rate of fertilizer, irrigation, intensive tillage, monoculture, use of chemical for pest control, improved soil and water conservation, improved natural resource management agronomic practices, improved value addition, improved livestock rearing and feeding, improved harvesting and post-harvest handling practices Abdul et al. [1].As sited by Paul [2] farm technology referred transformation of producers from using unproductive and endangered seed to productive variety, enabling farmers to reflect their role in demonstration and invent that enhances technology dissemination and shorten unnecessary resource wastage in the process. Thus, despite its prehistoric origins and hayseed caricature, farming is the source of Invention of all technology.
The study results confirmed that large efforts were made to increase agricultural productivity through technology dissemination in Ethiopia, but food insecurity remains a major challenge in the country. Improving smallholder’s productivity demands sustainable adoption of suitable and package-full agricultural technologies and practices. It also reported that the socio-economic factors plays detrimental role in agricultural technology transfer and dissemination; but little attention given for the role of social capital in technology adoption and its potential to create collective actions, reduce transaction costs, relax supply side constraints, and disseminate information Nizam et al. [3].
The study results revealed that the benefit of agricultural technology realized in enhancing production and productivity can be justified when yield increasing technologies are widely been demonstrated, disseminated and used. The agricultural technology adoption decision of farm households has been found to be function of irrigation use, land ownership right, security, credit access, distance to the nearest market, plot distance from the home stead, off-farm participation and tropical livestock unit Berihun et al. [4].The other result also implied that farmers who adopt technologies once are more likely to adopt the technologies due to its the profitability of agricultural technology adoption and agricultural extension services and technology adoption have a statistically significant and positive impact on nutrition and food security Tigabu and Gebeyehu [5].
Agricultural technology development and adoption is an essential approach for increasing agricultural productivity, achieving food self-sufficiency and alleviating poverty and food in-security among smallholder farmers in Ethiopia. In Ethiopia, farmers have been adopting and using different agricultural technologies at different proportion in relation to difference in Socioeconomic, institutional and environmental factors; the adoption of technologies has not completely optimal yet. Therefore, it is needed to further promote agricultural new technologies by designing based on farmer’s problem and demand. The article reviewed on the issue revealed that major explanatory variables significantly affect the adoption of agricultural new technologies by farmers comprised of age, education level, training and demonstration access, family size, tropical livestock unit, market distance, gender, farm size, improved infrastructure access, extension service provision and credit access Melesse, Leggesse et al. [6,7].
Empirical studies result on adoption of agricultural technologies in Ethiopia were concentrating on the adoption of fertilizer and improved seed varieties. However, the adoption rate of technologies by farm household is complex and interlinked that based on the type of technology and the time of study, lagged variables, socioeconomic and institutional factors. The farm households were adopting more of chemical fertilizers than improved seeds and their adoption rate increases for more recent studies Aynalem et el. [8].
The study result shows that younger farmers, famers with larger land size, farmer living closer to market, and farmers who had closer contact with the extension system are more likely to adopt new technology in better manner and proportion and use it more. The result underscores the need for research and extension programs to be sensitive to the needs of farmers when developing and disseminating technologies that are relevant to their agroecologies Admassie and Gezahegn [9].
The study results by Solomon et al. [10] show that knowledge of existing varieties, perception about the attributes of improved varieties; household wealth (livestock and land) and availability of active labour force are major determinants for adoption of improved technologies. The finding also suggests that the adoption of improved agricultural technologies has a significant positive impact on farmers integration into output market and the findings are consistent across the three models suggesting the robustness of the results Chilot et al. [11].
Doss et al. [12] found that extension was the variable most significantly affecting with wheat and maize technology adoption, and extension services continue to play an important role in disseminating information on new varieties and how to manage them.
Yohannes et al. [13] reported that economic factors such as income, wealth and debt family size, access to outside information, education and experience significantly known to influence on the adoption of pesticide technologies. The effect of socio-economic factors on adoption of fertilizer and pesticide technologies is greater in the area which has more access to outside information and offfarm activities than in more ‘self-contained’ area. The impact of the degree of risk aversion of farmers is found to be significant for fertilizer and pesticide technologies in only one area.
In General, the transfer and utilization of improved agricultural technologies depends on social, economic, environmental, institutional and demographic factors that all known to impose detrimental role in the system. However, the attention given to listed factors are uneven and related to these the speed of technology transfer and utilization was at stagnant position. In addition to these the agricultural constraints not listed in the way to generate information and policy formulation. Hence, the Meta analyses study in food crop technology at country level aimed for generation information over the technology adoption factors and the mean size effect of socioeconomic, institutional and demographic factors over adoption rate of food technologies.
Methodology for Meta-Analysis of Food Crop Technology Adoption
The methodology employed critical reviewed that supported by Meta-analysis of food crop technologies in Ethiopia. The major reviewed articles were the one that carried out in potential food crop production regions of Ethiopia such as Oromiya, SNNPR, Amhara and Tigray. The articles reviewed comprised that done from 1990 up to 2018.In the Meta-analysis, about 150 significantly influencing independent variables used that gathered from 48 different articles published and summarized to 48 observations from the Google. The articles reviewed categorized as cereal crop, pulse crop and root and tuber crops. The technologies defined for adoption food crop summarized consists of improved varieties, artificial fertilizer, chemicals for pest control and sowing method. The major food crops from which reviewed information gathered include wheat, maize, Teff and barley, from cereals, soybean, common bean and fababean from pulse crops, potato from tuber and root crops. From reviewed articles data created on excel and organized to see the mean size effect of commonly affecting variables over adoption of food crop technologies. The major data synthesized from reviewed articles include model type, publication date, year with author, data type, region the study done and sample size. The mean size effect independent variables selected based on its level of appearance at different articles. The explanatory variables gathered and synthesized for analysis comprised of gender, family size, age, education level, market distance and access, cooperative membership and distance, livestock ownership, TLU and oxen holding, training, participation in field day, market access for input and output market, market distance, land size and plot distance from homestead, price perception, risk and nutrient perception, road access and distance, artificial fertilizer used, irrigation access, seed availability, fertility, extension access and contacts, income, machinery rent, fertility, district and radio ownership.Steps followed in analyzing data in Meta-Analysis for food crop technology adoption
• Calculating Standard Error (SE)All Standard errors estimates derived from the formula se=


• Computing variance (Var)
This formula is simple: Var = SE2. In Excel it was calculated by just squaring the estimated standard error, Var=squarer of Standard errors.
• Computing individual study weights (w)
The weight estimated have to to be estimated that weight each study with the inverse of its by dividing 1 by its variance, so the weight variance calculated as
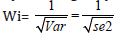
• Computing each weighted effect size (w*es)
coefficients by the study weight. Therefore WiYi=Wi*Yi, where Wi is the weighted effect size and Yi the value of coefficients over study results.
• Other necessary variables (w*es2 and w2 and M*)
These two important variables estimated by just multiplying the calculated results. This is to mean that w*es and w2. From these values the Mean size effect calculated by the formula

• Calculating Variance, Standard Error and t value
The variance can be calculated by



• Empirical model
The empirical model of the meta-analysis regressed using Multinomial logit model procedure. The empirical model considered adoption rate of food crop technology as dependant variable and study region, model type used, data type, food crop technology type used, study period, sample size as explanatory variables. The adoption probability estimate of the specific technology defined as function of sample size, model used, data type, year of publications and region where the survey done, The mathematical expressions of defining the adoption rate function can be written as:

Where n is the sample size for the specific study, x1 is the model type (defined as 1 if Probit and 0 otherwise, x2 is data type used (defined as 1 if cross sectional and 0 otherwise) ,x3 is the year of the publications (labelled as 1 if the study undertaken after 2010 and 0 otherwise),x4 referred to the region where the study done (labelled as 1 if Oromiya and 0 otherwise).
Critical article Review and synthesizing on food crop technology adoption
Review in technology adoption of cereal crops in Ethiopia: The study report by revealed that when the farmers access fertilizer and seed their access to extension services seemed automatic and that production specialization together with wealth play a major role in explaining crop area under fertilizer and improved seed for cereal crop production Bingxin et.al. [14]. Extension service, risk aversion behaviour, wealth and land fragmentation played detrimental role in adoption of fertilizer in cereal crop production Bingxin and Alejandrot [15].Practical training has positive and significant effects on the likelihood of adopting improved varieties of barley; secondary level education has positive and significant effects on the likelihood of adopting improved varieties of barley and wheat. Hence, efforts to increase adoption of improved barley and wheat varieties would more likely be successful if accompanied with practical trainings and demonstrations Chilot et al. [16].
Medkissa et al. [17] indicated that for the strength of wheat production system, the existences of strong farmers-extensionresearch linkage among actors within the system has a vital importance in a way that to transfer skill, knowledge and provision of improved bread wheat varieties in efficient and effective manner is mandatory.
The empirical study result by Tesfaye et al. [18] showed that the existence of supportive relationship between household head sex, field day participation, roads access, and district potentiality in enhancing the adoption of improved wheat varieties. The level of improved wheat varieties was also known to be inverse function of household head gender and road access to all weather roads. The level adoption of improved wheat varieties is defined as function of access to credit, active family force, market distance and district potentiality. The overall findings of the study emphasized household head sex, field day participation, access to all weather roads, access to credit, active family force, district and market distance as being key determinants on the intensity and adoption of use of improved wheat varieties.
The farmer’s total wheat area, number of livestock, and the use of hired labour and credit significantly influenced the amount of fertilizer used. The age of the farmer, the use of credit, and several varietal characteristics preferred by farmers (disease and lodging resistance and baking quality) significantly influenced the area allocated to improved wheat varieties Bekele et al. [19].
The use of local and low-yielding wheat varieties, incidence of diseases and pests, poor agronomic practices and declining soil fertility have been identified as major constraints to low wheat production and productivity in Ethiopia. Household level human capital, household asset endowment, farm level institutional factors and policy variables significantly affect the raw planting technology adoption of Teff production in Southern Ethiopia Geremew et al. [20].
The study report revealed that household being headed education level, family size, Farm size, annual off-farm income, distance to nearest market and training on row planting significantly influenced adoption and level of adoption of row planting. Moreover, adoption of row planting is significantly affected by Farming experience, No. of information sources and distance to Farmers training centres whereas level of adoption of row planting by livestock (TLU) and Number of oxen Tafesse [21]. Joachim et al. [22] found that row planting for Teff yields higher productivity at demonstration than at large scale area of production due to implementation problem in recombined agronomic practices.
The survey result revealed that farm size, household income, access to credit, contact with extension agents, participation in training, and field day were positively and significantly influenced whereas, age of household and market distance negatively influenced adoption and intensity of use of improved highland maize varieties production in the study area Dawit and Abduselam [23].The study result revealed that difference in production district, labour force, membership to cooperatives, distance to FTC, and livestock holding significantly affected smallholders’ intensity of adoption of DAP in maize production Ketema and Kebede [24].
The survey result in eastern Ethiopia indicated that there are positive relationships between improved seed and fertiliser and between improved seed and soil conservation. There were also negative relationships between adoption of manure and fertiliser and between manure and improved seed. The estimation results indicated that the variables affecting farmers’ decisions to adopt a technology differ between technologies. Educational level of the household, family size, off /non activities, livestock ownership, and distance to the market, plot ownership, slop of the plot and other variables also play significant roles, partly with differing signs across technologies Musa [25].
The study result by Bedru and Dagne [26] indicated that Adoption of hybrid maize varieties depends on ages, education level, farm size and land proportion allocated to specific crop, extension service, market distance and altitude of the district. Bedru et al. [27] expressed that farmland size positively influences the adoption of improved OPVs of maize. On the other hand, the adoption decision of improved OPVs is negatively influenced by distance from the nearest grain market. The frequency of substantial yield stress encountered due to drought is an impediment to adoption of improved OPVs of maize and is of concern for mitigation through adoption of improved drought tolerant maize varieties. Abadi et al. [28] confirmed that adoption of the improved maize varieties among Maize producer households was found to be positively influenced by adult-literacy, family size, livestock wealth, access to output market and credit access for the new varieties. On the other hand, farmer associations, distance to main markets and fertilizer credit negatively influenced adoption.
Ketema and Kebede [29] indicated by study result revealed that variation in districts, family size, membership to cooperatives, distance to farmers training centre, and livestock holding significantly affected smallholders’ intensity of adoption of DAP in maize production. On the other side, variation in district, farming experience, farm size, membership to cooperatives, dependency ratio, and annual income significantly determined intensity of adoption of Urea. Tesfaye and Alemu [30] found that level of education and family size were found to significantly and positively influence die adoption decision of improved maize and chemical fertilizer. Different maize varieties have different productivity at due to different in potentiality across districts Musba [31].
Factors affecting the adoption rate and intensity and utilization of the improved sorghum varieties across their suitable agro ecology summarized as extension service, access to improved varieties and its production packages, training in form of awareness creation and field day and access to market points for farmers Kinfe [32].
Review of Root and tuber crop technology adoption:Although potato is one of the strategic crops for ensuring food security in Ethiopia, the adoption of high yielding and disease tolerant improved potato varieties is low. Higher education of the household head and the presence of a radio and/or television also have a positive effect on adoption. As to the scale of adoption, we found that only the percentage of owned land, tuber size (of ware potatoes), access to credit, stew quality, and presence of a mobile phone have an impact on ware potato farmers’ decision on the amount of land to be used for growing Improved Varieties Gumataw et al. [33].
Ketema et al. [34] confirmed that Variation in districts, access to irrigation, farm size, membership to cooperatives, and annual income of the households were found to significantly affect the adoption of potato technology package. Kebede and Ketema [34] study result revealed that variation in districts, access to irrigation, frequency of extension contact, and livestock holding significantly affected intensity of adoption of both DAP and Urea in potato production.
Review on adoption of pulse crops
The study by Alemneh et al. [35] showed that the household head educational status, age, land size and household size affect consumption and production of pulse crops. The survey result by Chilot et al. pointed out that practical training has positive and significant effects on the likelihood of adopting improved varieties of fababean. Hence, efforts to increase adoption of improved agronomic practices and varieties would more likely be successful if accompanied with practical trainings and access to improved knowledge.
Hagoes et al. [36] study result revealed that the decision to adopt improved fababean varieties is positively influenced by annual farm income and training obtained but negatively influenced by residents of the household heads. The study concludes that adoption decision was found to be a combination of economic, physical and institutional variables of the farmers.
The survey results show that knowledge of existing varieties, perception about the attributes of improved varieties, livestock and land ownership and availability of active family labour force play a significant role in enhancing the level of adoption of improved chickpea varieties. The report also confirmed that adoption of improved agricultural technologies has a significant positive impact on marketed surplus and the findings are consistence across the three models suggesting the robustness of the results. Integration into output market is also positively associated with household wealth and availability of active family labour force and negatively associated with age of household head and distance to main market Solomon et al. [37].
The decision to adopt white haricot beans variety is known to positively supported by frequency of extension visits, land size allocated to haricot beans, agricultural income, price perception, training obtained and perception on fertility enhancement benefit of the crop, and negatively by distant to market, ownership of haricot beans farm land (ten and nutritional perception of the crop. The intensity of adoption of white beans is affected negatively by the number of dependents in the household, ownership of haricot beans land (tenure) and positively by non-farm income and contact with non-governmental organizations Daniel et al. [38].
The survey result by Yitayal and Adam [39] revealed that that speed of adoption of beans better at male headed households compared to female headed households and the size of cultivated land, proximity to extension office and remoteness to fertilizer market hastened adoption, while dependency ration and livestock ownership contributed to accelerated in the adoption probability.
The study result of various findings indicated that household head’s attitude towards common bean production technology package, participation in extension event (participation in training and field visit) and access to credit were important variables which had positively and significantly influenced adoption and intensity of adoption of improved common bean production package. Whereas, perceived relative is advantage of technology attributes of the household head had shown negative relationship with adoption and intensity of adoption. Some farmers who previously adopted improved common bean varieties have discontinued planting the varieties mainly due to market problem and poor management practice Amanuel and Girma [40].
The study report indicated that existence of the variation in adoption of improved varieties that implies difference in access to inputs and information about the improved varieties. At present input supply (especially seed) is limited or non-existent particularly in the remote chickpea producing areas EARO [41]. The study result by Samuel et al. [42] showed that attendance on training of soybean production and use of soy food at home affects soybean adoption positively and significantly. However, age of the household head and distance to main market has negative and significant impact.
Review on adoption of artificial fertilizer
The survey results indicated existence of positive impact of extension and credit services, age, farmland size, education, livestock, off/non-farm income and gender in enhancing the adoption of inorganic fertilizer. Physical characteristics like distance from farmers’ home to markets, roads, credit and input supply played a critical role in the adoption of inorganic fertilizers as proximity to information, sources of input and credit supply and markets save time and reduce transportation costs Hassen et al. [43].
The study showed adoption of DAP fertilizer depends on location variables, farmer type, institutional factors and resource endowment. The study revealed that farmers residing in the SNNP cluster and Eastern and Western Oromiya apply significantly less DAP fertilizer to cereal crops as compared to farmers dwelling in Central Oromiya. On the contrary farmers in the Bahir Dar cluster apply significantly higher levels of DAP fertilizer as compared to farmers in central Oromiya. Model farmers are more likely to apply a higher amount of DAP fertilizer to cereal crops as compared to non-model farmers. Access to credit and cooperatives membership were the two institutional factors that were found to positively influence the level of DAP fertilized applied to cereal crops. The tropical livestock units and annual gross income significantly affected the adoption decision and level of DAP Tewodros et al. [44].
The study results showed that the decisions to use fertilizer and manure are negatively related to one another. Fertilizer is expensive in prices and inadequate in supply but less demanding of labour in its application. The parcel size has a positive effect on both land management practices. Access to credit is found to be significant in positively affecting the probability of using fertilizer. This implies that credit is very helpful in relieving capital constraints faced by farmers for using fertilizer and other purchased inputs Ketema and Siegfried [45].The use and intensity of adoption of fertilizer defined as function of age of the farmer, farmers’ expectations of rainfall conditions and farmers’ perception of the price of fertilizer Fufa and Hassan [46].
Meta-analysis research questions for food Crop technology adoption
i. What were the major food crop technologies had been transferred in agricultural sector in Ethiopia.ii. Do differences exist in relation to proportion of technology adoption probability across study periods, data type used, region, model employed, and sample size used.
iii. Which small scale farmer socio-economic, institutional and demographic factors that significantly influences mean size effect of probability of food crop technology adoption.
Results and Discussion
The estimated LR 159 is highly statistically significant, its p value being practically zero. This points out that the model we have chosen gives a good fit. This suggests that the model we have chosen gives a good fit, although not every slope coefficient is statistically significant. This also justified that the regressors have strong influence on the choice probability (Table 1).Table 1: Model Fitting Information.

The table above confirmed that the independent variables included in the model significantly affected the dependent variable. Hence, it recommended for further analysis and interpretation of results based on results estimated. The mode fitness also checked and confirmed by estimates Pseudo R-Square. From the estimates indicated by Negelkerke calculated value that the included explanatory variable explained 60% of Model estimate (Table 2).
To read more about this article... Open access Journal of Nutrition & Food Science
Please follow the URL to access more information about this article
To know more about our Journals....Iris Publishers
To know about Open Access Publishers
No comments:
Post a Comment